Application-Specific Integrated Circuit (ASIC) Miner
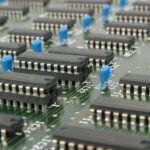
[ad_1]
What Is an Application-Specific Integrated Circuit (ASIC) Miner?
An application-specific integrated circuit (ASIC) is an integrated circuit chip designed for a specific purpose. An ASIC miner is a computerized device that uses ASICs for the sole purpose of “mining” digital currency. Generally, each ASIC miner is constructed to mine a specific digital currency. So, a Bitcoin ASIC miner can mine only bitcoin. One way to think about bitcoin ASICs is as specialized bitcoin mining computers optimized to solve the mining algorithm.
Developing and manufacturing ASICs as mining devices is costly and complex. However, because ASICs are built especially for mining cryptocurrency, they do the job faster than less powerful computers. As a result, ASIC chips for cryptocurrency mining have become increasingly efficient, with the latest generation hashing at 158 terahashes per second but only using 34.5 joules per terahash.
Key Takeaways
- An application-specific integrated circuit (ASIC) miner is a computerized device that uses ASICs for the sole purpose of mining bitcoin or another cryptocurrency.
- An application-specific integrated circuit (ASIC) is generally optimized to compute just a single function or set of related functions.
- Bitcoin miners review and verify previous bitcoin transactions and create new blocks to add the data to the blockchain.
Understanding Application-Specific Integrated Circuit (ASIC) Miners
Instead of being general-purpose integrated circuits—like RAM chips or PC or mobile device microprocessors—ASICs employed in cryptocurrency mining are specific integrated circuits designed solely to mine cryptocurrencies.
Initially, Bitcoin’s creator(s) intended for bitcoin to be mined on central processing units (CPUs) of commonly used laptops or desktop computers. However, Bitcoin ASICs surpassed both CPUs and graphics processing units (GPUs) because of their reduced electricity consumption and greater computing capacity. After gaining traction in mid-2013, when other hardware mining devices started hitting bottlenecks in their mining, Bitcoin ASIC miners increased and retained their lead.
Contrary to popular belief, mining is not complex mathematical computation. It is the process of changing few numbers on a hash find one that is less than the target hash (the original hash).
A hash is a long hexadecimal number used to identify blocks in a blockchain, called the block header hash or block hash. To mine a block, miners begin adding values to a hash to generate new ones until a number less than the target difficulty (original hash) is reached. This is called hashing. The more hashes that can be performed in a set period, the more likely a miner is to earn bitcoin. ASIC miners are optimized to compute hash functions efficiently and quickly.
Although mining cryptocurrencies can be an expensive proposition of declining profitability, many people are drawn to it. Despite the uncertain return on investment, would-be cryptocurrency miners are willing to incur high upfront expenses for pricey ASICs and pay significant ongoing costs for electricity in return for the prospect of earning cryptocurrency.
Development of the ASIC Miner
Cryptocurrency mining is required by a proof of work (PoW) blockchain like Bitcoin to carry out its operations. The mining process involves solving a block’s hash by randomly generating numbers until reaching a number below the target difficulty number. The first miner to find the solution to the puzzle closes the block. Each winner in the bitcoin mining competition receives a reward (a specific amount of bitcoin) along with the transaction fees for the transactions in that block.
In Bitcoin’s early days, any computer with adequate processing power could mine bitcoin. However, those days are long gone; bitcoin’s soaring popularity and growing acceptance have attracted hordes of crypto miners.
At the same time, cryptocurrency mining has become exponentially more difficult because the mining difficulty changes as miners enter and exit the network. Over time, the number of miners has constantly grown, which increased the difficulty. These developments have resulted in a race to harness the most “hashing power,” the term used to describe how many hashes per second a miner can generate (or the combined hashes per second of a networked mining rig or pool). ASIC miners came about as a result of this quest for more hashing power; modern Bitcoin ASICs can hash at more than 150 terahashes per second (nine zeros, or 150 x 1012 hashes per second).
ASIC devices were popularized by Bitmain (headquartered in China), which dominates ASIC Bitcoin mining activities through its Antminer ASIC product range.
ASIC Miner Advantages
Though GPU and CPU mining rigs rely on components that have more than one function, ASIC miners are designed for the sole purpose of mining cryptocurrency. This singular focus makes an ASIC miner much more powerful and energy-efficient than a comparable GPU miner.
Because each cryptocurrency has its own cryptographic hash algorithm, an ASIC miner is designed to mine using that specific algorithm. For example, Bitcoin ASIC miners are designed to hash the SHA-256 algorithm, while Litecoin (LTC) uses scrypt (pronounced es-crypt). Though this means that an ASIC miner could technically mine any other cryptocurrency based on the same algorithm, most miners who invest in ASIC hardware designed to mine bitcoin or Litecoin stick to mining that specific cryptocurrency.
Many miners join a mining pool to increase their chances of earning bitcoin. Mining pools usually pay shares of rewards based on a miner’s hashrate and work contributed.
ASIC Miner Considerations
Before investing thousands of dollars in an ASIC mining rig, here are some factors to be considered:
- What coins can be mined? The list of cryptocurrencies that can be mined with ASICs is far smaller than those that can be mined with a GPU rig. Cryptocurrencies that can be mined with ASICs include Bitcoin, Litecoin, and several others.
- Rig location: Though GPU mining rigs can be located in one’s home, ASIC miners are louder and generate much more heat. This means that one’s home is not ideal for an ASIC miner, and alternate locations like a basement or garage with cooling need to be considered.
- Power consumption: The latest generation of ASIC machines are more energy-efficient than GPU rigs but consume tremendous power nevertheless. An ASIC miner based in one’s home may necessitate upgrading the electrical wiring system to handle the increased power load.
- Choosing a Bitcoin mining pool: Mining pools enable miners to combine the power of their ASIC miner rigs to mine bitcoin and share the rewards for successfully minted blocks. Factors to be considered when choosing a pool include its reputation, size, and payment rules.
- Return on Investment: Is the return on investment sufficiently high enough to justify the upfront cost of an ASIC miner and ongoing operating expenses?
What Is Bitcoin Mining?
Bitcoin mining is the process of solving for the two-digit encrypted number contained in a block’s hash called the nonce. A miner adds values (the nonce) to a block’s hash trying to generate a number less than the difficulty target. When it is solved, the hash is solved, and the block is validated. The validator receives a reward.
What Is the Difference Between ASIC Mining and GPU Mining?
ASIC mining machines are developed for mining a specific cryptocurrency, such as Bitcoin or Litecoin. GPU mining involves using a graphics processing unit (GPU) such as those sold by NVIDIA or AMD for mining. GPUs are significantly cheaper than the equipment required for ASIC mining. However, they are slower and much less efficient for mining cryptocurrencies than ASIC miners.
What Are ASIC-Resistant Coins?
ASIC-resistant coins are cryptocurrencies with ASIC-resistant algorithms. Mining these cryptocurrencies with ASIC mining equipment is virtually impossible; even if one tries to do so, the returns would be limited. The primary rationale for ASIC-resistant coins is to preserve the decentralization of their blockchains, which was one of the core principles behind creating Bitcoin.
[ad_2]
Source link