What Is an Actuarial Gain Or Loss? Definition and How It Works
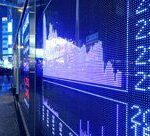
[ad_1]
What is an Actuarial Gain Or Loss?
Actuarial gain or loss refers to an increase or a decrease in the projections used to value a corporation’s defined benefit pension plan obligations. The actuarial assumptions of a pension plan are directly affected by the discount rate used to calculate the present value of benefit payments and the expected rate of return on plan assets. The Financial Accounting Standards Board (FASB) SFAS No. 158 requires the funding status of pension funds to be reported on the plan sponsor’s balance sheet. This means there are periodic updates to the pension obligations, the fund performance and the financial health of the plan. Depending on plan participation rates, market performance and other factors, the pension plan may experience an actuarial gain or loss in their projected benefit obligation.
While those accounting rules require pension assets and liabilities to be marked to market on an entity’s balance sheet, they allow actuarial gains and losses, or changes to actuarial assumptions, to be amortized through comprehensive income in shareholders’ equity rather than flowing directly through the income statement.
Key Takeaways
- Actuarial gains and losses are created when the assumptions underlying a company’s projected benefit obligation change.
- Accounting rules require companies to disclose both the pension obligations (liabilities) and the assets meant to cover them. This shows investors the overall health of the pension fund.
- All defined benefits pension plans will see periodic actuarial gains or losses as key demographic assumptions or key economic assumptions making up the model are updated.
Understanding Actuarial Gain Or Loss
Actuarial gains and losses are best understood in the context of overall pension accounting. Except where specifically noted, this definition addresses pension accounting under U.S. generally accepted accounting principles (GAAP). While U.S. GAAP and International Financial Reporting Standards (IFRS) prescribe similar principles measuring pension benefit obligations, there are key differences in how the two standards report pension cost in the income statement, particularly the treatment of actuarial gains and losses.
Funded status represents the net asset or liability related to a company’s defined benefit plans and equals the difference between the value of plan assets and the projected benefit obligation (PBO) for the plan. Valuing plan assets, which are the investments set aside for funding the plan benefits, requires judgment but does not involve the use of actuarial estimates. However, measuring the PBO requires the use of actuarial estimates, and it is these actuarial estimates that give rise to actuarial gains and losses.
There are two primary types of assumptions: economic assumptions that model how market forces affect the plan and demographic assumptions that model how participant behavior is expected to affect the benefits paid. Key economic assumptions include the interest rate used to discount future cash outflows, expected rate of return on plan assets and expected salary increases. Key demographic assumptions include life expectancy, anticipated service periods and expected retirement ages.
Actuarial Gains and Losses Create Volatility in Results
From period to period, a change in an actuarial assumption, particularly the discount rate, can cause a significant increase or decrease in the PBO. If recorded through the income statement, these adjustments potentially distort the comparability of financial results. Therefore, under U.S. GAAP, these adjustments are recorded through other comprehensive income in shareholders’ equity and are amortized into the income statement over time. Under IFRS, these adjustments are recorded through other comprehensive income but are not amortized into the income statement.
Footnote Disclosures Contain Useful Information About Actuarial Assumptions
Accounting rules require detailed disclosures related to pension assets and liabilities, including period-to-period activity in the accounts and the key assumptions used to measure funded status. These disclosures allow financial statement users to understand how a company’s pension plans affect financial position and results of operations relative to prior periods and other companies.
[ad_2]
Source link