Average Daily Rate (ADR): Definition, Calculation, Examples
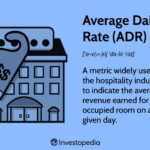
[ad_1]
What Is the Average Daily Rate (ADR)?
The average daily rate (ADR) is a metric widely used in the hospitality industry to indicate the average revenue earned for an occupied room on a given day. The average daily rate is one of the key performance indicators (KPI) of the industry.
Another KPI metric is the occupancy rate, which when combined with the ADR, comprises revenue per available room (RevPAR), all of which are used to measure the operating performance of a lodging unit such as a hotel or motel.
Key Takeaways
- The average daily rate (ADR) measures the average rental revenue earned for an occupied room per day.
- The operating performance of a hotel or other lodging business can be determined by using the ADR.
- Multiplying the ADR by the occupancy rate equals the revenue per available room.
- Hotels or motels can increase the ADR through price management and promotions.
Understanding the Average Daily Rate (ADR)
The average daily rate (ADR) shows how much revenue is made per room on average. The higher the ADR, the better. A rising ADR suggests that a hotel is increasing the money it’s making from renting out rooms. To increase the ADR, hotels should look into ways to boost price per room.
Hotel operators seek to increase ADR by focusing on pricing strategies. This includes upselling, cross-sale promotions, and complimentary offers such as free shuttle service to the local airport. The overall economy is a big factor in setting prices, with hotels and motels seeking to adjust room rates to match current demand.
To determine the operating performance of a lodging, the ADR can be measured against a hotel’s historical ADR to look for trends, such as seasonal impact or how certain promotions performed. It can also be used as a measure of relative performance since the metric can be compared to other hotels that have similar characteristics, such as size, clientele, and location. This helps to accurately price room rentals.
Calculating the Average Daily Rate (ADR)
The average daily rate is calculated by taking the average revenue earned from rooms and dividing it by the number of rooms sold. It excludes complimentary rooms and rooms occupied by staff.
Average Daily Rate=Number of Rooms SoldRooms Revenue Earned
Example of the Average Daily Rate (ADR)
If a hotel has $50,000 in room revenue and 500 rooms sold, the ADR would be $100 ($50,000/500). Rooms used for in-house use, such as those set aside for hotel employees and complimentary ones, are excluded from the calculation.
Real World Example
Consider Marriott International (MAR), a major publicly traded hotelier that reports ADR along with occupancy rate and RevPAR. For 2019, Marriott’s ADR increased by 2.1% from 2018 to $202.75 in North America. The occupancy rate was fairly static at 75.8%. Taking the ADR and multiplying it by the occupancy rate yields the RevPAR. In Marriott’s case, $202.75 times 75.8% equates to a RevPAR of $153.68, which was up 2.19% from 2018.
The Difference Between the Average Daily Rate (ADR) and Revenue Per Available Room (RevPAR)
The average daily rate (ADR) is needed to calculate the revenue per available room (RevPAR). The average daily rate tells a lodging company how much they make per room on average in a given day. Meanwhile, RevPAR measures a lodging’s ability to fill its available rooms at the average rate. If the occupancy rate is not at 100% and the RevPAR is below the ADR, a hotel operator knows that it can probably reduce the average price per room to help increase occupancy.
Limitations of Using the Average Daily Rate (ADR)
The ADR does not tell the complete story about a hotel’s revenue. For instance, it does not include the charges a lodging company may charge if a guest does not show up. The figure also does not subtract items such as commissions and rebates offered to customers if there is a problem. A property’s ADR may increase as a result of price increases, however, this provides limited information in isolation. Occupancy could have fallen, leaving overall revenue lower.
[ad_2]
Source link