Autonomous Expenditure
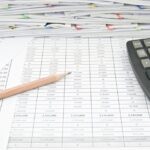
[ad_1]
What is an Autonomous Expenditure?
An autonomous expenditure describes the components of an economy’s aggregate expenditure that are not impacted by that same economy’s real level of income. This type of spending is considered automatic and necessary, whether occurring at the government level or the individual level. The classical economic theory states that any rise in autonomous expenditures will create at least an equivalent rise in aggregate output, such as GDP, if not a greater increase.
Understanding Autonomous Expenditure
An autonomous expenditure obligation must be met regardless of income. It is considered independent in nature, as the need does not vary with incomes. Often, these expenses are associated with the ability to maintain a state of autonomy. Autonomy, in regard to nations, includes the ability to be self-governing. For individuals, it refers to the ability to function within a certain level of societally acceptable independence.
To be considered an autonomous expenditure, the spending must generally be deemed necessary to maintain a base level of function or, in an individual sense, survival. Often, these expenses do not vary regardless of personal disposable income or national income. Autonomous expenditure is tied to autonomous consumption, including all of the financial obligations required to maintain a basic standard of living. All expenses beyond these are considered part of induced consumption, which is affected by changes in disposable income.
In cases in which personal income is insufficient, autonomous expenses still must be paid. These needs can be met through the use of personal savings, consumer borrowing mechanisms such as loans and credit cards, or various social services.
Key Takeaways
- Autonomous expenditures are expenditures that are necessary and made by a government, regardless of the level of income in an economy.
- Most government spending is considered autonomous expenditure because it is necessary to run a nation.
- Autonomous expenditures are related to autonomous consumption because they are necessary to maintain a basic standard of living.
- External factors, such as interest rates and trade policies, affect autonomous expenditures.
Autonomous Expenditures and Income Levels
While the obligations that qualify as autonomous expenditures do not vary, the amount of income directed toward them can. For example, in an individual sense, the need for food qualifies as an autonomous expenditure, though the need can be fulfilled in a variety of manners, ranging from the use of food stamps to eating every meal at a five-star restaurant. Even though income level may affect how the need is met, the need itself does not change.
Governments and Autonomous Expenditures
The vast majority of government spending qualifies as autonomous expenditures. This is due to the fact that the spending often relates strongly to the efficient running of a nation, making some of the expenditures required in order to maintain minimum standards.
Factors Affecting Autonomous Expenditures
Technically, autonomous expenditures are not affected by external factors. In reality, however, several factors can affect autonomous expenditures. For example, interest rates have a significant effect on consumption in an economy. High interest rates can tamp down on consumption while low interest rates can spur it. In turn, this affects spending within an economy.
Trade policies between countries can also affect autonomous expenditures made by their citizens. If a producer of cheap goods imposes duties on exports, then it would have the effect of making finished products for outside geographies more expensive. Governments can also impose controls on an individual’s autonomous expenditures through taxes. If a basic household good is taxed and no substitutes are available, then the autonomous expenditure pertaining to it may decrease.
Examples of Autonomous Expenditure
Some of the spending classes that are considered independent of income levels, which can be counted as either individual income or taxation income, are government expenditures, investments, exports, and basic living expenses such as food and shelter.
[ad_2]
Source link